
ERROR - Page not foundThe web page you requested was not found.Some possible reasons for this:
Please use the menu at the left side of the page or the search at the top of the page to find what you are looking for. If you can't find the information you need please contact the webmaster |
Find more about:
Jump to:
Undergraduate Courses |
Graduate Courses |
Events Newsletter |
Outreach Activities |
Observatory Nights |
Alumni & Friends |
Alumni Newsletter |
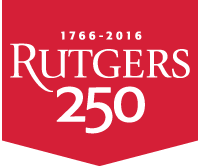